Motion as Emotion
Motion as Emotion is a project that explored the use of free-hand gestures in VR as a means of understanding user affect and cognitive load. It was inspired by the release of the Vision Pro, which demonstrated the commercial feasibility of XR interfaces that primarily use eye gaze and free-hand gestures as input, as well as by prior work demonstrating that user inputs such as keystroke patterns and mouse movements contain affective information.
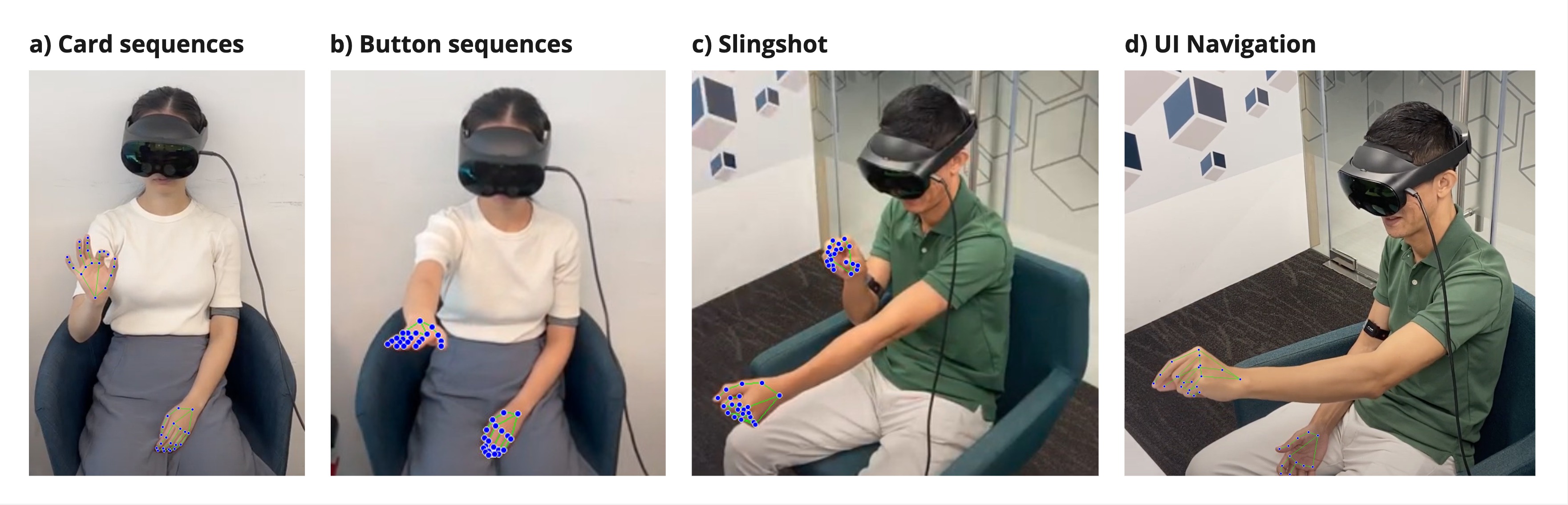
We conducted a user study with 22 participants, and designed several tasks with varying levels of difficulty in VR to elicit stress, cognitive load and feelings of fun/enjoyment. We draw on prior work to break down each gesture into phases such as preparation (moving the hands away from a resting position), pre-stroke hold, and stroke (the movement trajectories used to indicate a command). Then, we characterize the differences in features such as gesture distance and speed, hand tension, and head motion during these gesture phases while participants are engaging in the VR tasks.
We find significant differences in many of these features between the easy and difficult versions of the VR tasks. Standard support vector classification models were able to predict two levels (low, high) of arousal, valence and cognitive load from these features with very minimal fine-tuning.
A key advantage of the approach outlined in the paper is that it leverages data that is already available from most standard XR headsets (hand and head-motion tracking data), enabling recognition of affective and congitive states without needing any additional wearable sensors.
Role
For this project, I led the conceptualization, study design and data analysis.
Publications
Motion as Emotion: Detecting Affect and Cognitive Load from Free-Hand Gestures in VR
Under review for CHI 2025
Phoebe Chua, Prasanth Sasikumar, Yadeesha Weerasinghe, Suranga Nanayakkara